Learning an Intrinsic Garment Space
for Interactive Authoring of Garment Animation
Tuanfeng Y. Wang1 Tianjia Shao2 Kai Fu1 Niloy Mitra3
1miHoYo Inc.
2University of Leeds
3University College London
Siggraph Asia 2019
Abstract
Authoring dynamic garment shapes for character animation on body motion is one of the fundamental steps in the CG industry. Established workflows are either time and labor consuming (i.e., manual editing on dense frames with controllers), or lack keyframe-level control (i.e., physically-based simulation). Not surprisingly, garment authoring remains a bottleneck in many production pipelines. Instead, we present a deep-learning-based approach for semi-automatic authoring of garment animation, wherein the user provides the desired garment shape in a selection of keyframes, while our system infers a latent representation for its motion-independent intrinsic parameters (e.g., gravity, cloth materials, etc.). Given new character motions, the latent representation allows to automatically generate a plausible garment animation at interactive rates. Having factored out character motion, the learned intrinsic garment space enables smooth transition between keyframes on a new motion sequence. Technically, we learn an intrinsic garment space with an motion-driven autoencoder network, where the encoder maps the garment shapes to the intrinsic space under the condition of body motions, while the decoder acts as a differentiable simulator to generate garment shapes according to changes in character body motion and intrinsic parameters. We evaluate our approach qualitatively and quantitatively on common garment types. Experiments demonstrate our system can significantly improve current garment authoring workflows via an interactive user interface. Compared with the standard CG pipeline, our system significantly reduces the ratio of required keyframes from 20% to 1 − 2%.
Video
Motion Invariant Encoding
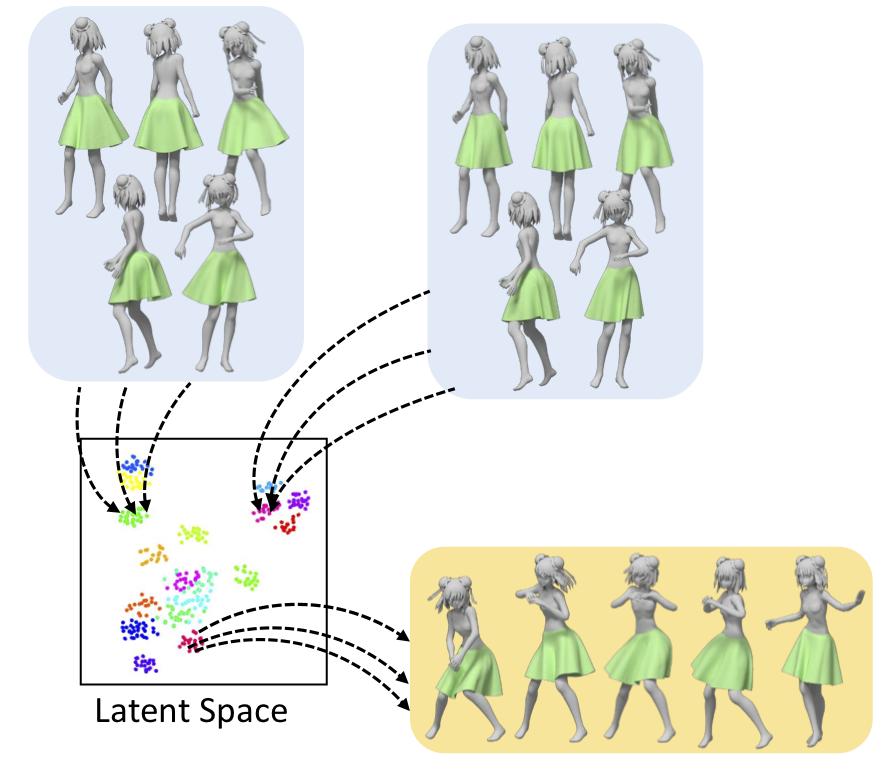
We train a motion invariant encoding that maps the garment shapes with the same intrinsic parameters to the same location in the latent space, while factorizing the motion of the underlying character, and decodes a location in the latent space to various garment shapes using the provided character’s motions. In the latent space, dots with the same color represent instances generated by the same intrinsic parameters.
Free Shape Generation

Here is a visual diagram of our motion invariant encoding network as proposed in our paper. We use a learned motion descriptor as the coefficients to linearly blend the multiple sub-networks within each network layer to dynamically change the network behavior according to the motion.
Bibtex
@article{WangEtAl:GarmentAuthoring:SiggraphAsia:2019 title={Learning an Intrinsic Garment Space for Interactive Authoring of Garment Animation}, author={Wang, Yangtuanfeng and Shao, Tianjia and Fu, Kai and Mitra, Niloy}, journal={ACM Trans. Graph.}, volume = {38}, number = {6}, artical = {220}, doi = {10.1145/3355089.3356512} }
Acknowledgements
We thank the reviewers for their comments and suggestions for improving the paper. The authors would also like to thank Zhiqing Zhong, Qi Tang, Jun Qian for making test cases for our system and Fox Dong for the video voice-over. This work is in part supported by miHoYo Inc., an ERC Starting Grant (SmartGeometry StG-2013-335373), ERC PoC Grant (SemanticCity), Google Faculty Award, Royal Society Advanced Newton Fellowship, gifts from Adobe, the New Faculty Start-Up Grant of University of Leeds, and NSF of China (No. 61772462, No. U1736217).