Deep Detail Enhancement for Any Garment
Meng Zhang1 Tuanfeng Wang2,3 Duygu Ceylan2 Niloy J. Mitra1,2
1University College London 2 Adobe Research
3Mihoyo Inc.
Eurographics 2021
Abstract
Creating fine garment details requires significant efforts and huge computational resources. In contrast, a coarse shape may be easy to acquire in many scenarios (e.g., via low-resolution physically-based simulation, linear blend skinning driven by skeletal motion, portable scanners). In this paper, we show how to enhance, in a data-driven manner, rich yet plausible details starting from a coarse garment geometry. Once the parameterization of the garment is given, we formulate the task as a style transfer problem over the space of associated normal maps. In order to facilitate generalization across garment types and character motions, we introduce a patch-based formulation, that produces high-resolution details by matching a Gram matrix based style loss, to hallucinate geometric details (i.e., wrinkle density and shape). We extensively evaluate our method on a variety of production scenarios and show that our method is simple, light-weight, efficient, and generalizes across underlying garment types, sewing patterns, and body motion.
Video
Pipeline

Given a coarse garment geometry S represented as a 2D normal map N, we present a method to generate an enhanced normal map N* and the corresponding high resolution geometry S*. At the core of our method is a detail enhancement network that enhances local garment patches in N conditioned on the material type \theta of the garment. We combine such enhanced local patches to generate N* which captures fine wrinkle details. We lift N* to 3D to generate S* by an optimization method that avoids interpenetrations between the garment and the underlying body surface. In case the garment material is not known in advance, we present a material classification network that operates on the local patches cropped from the coarse normal map N.
Base training Data
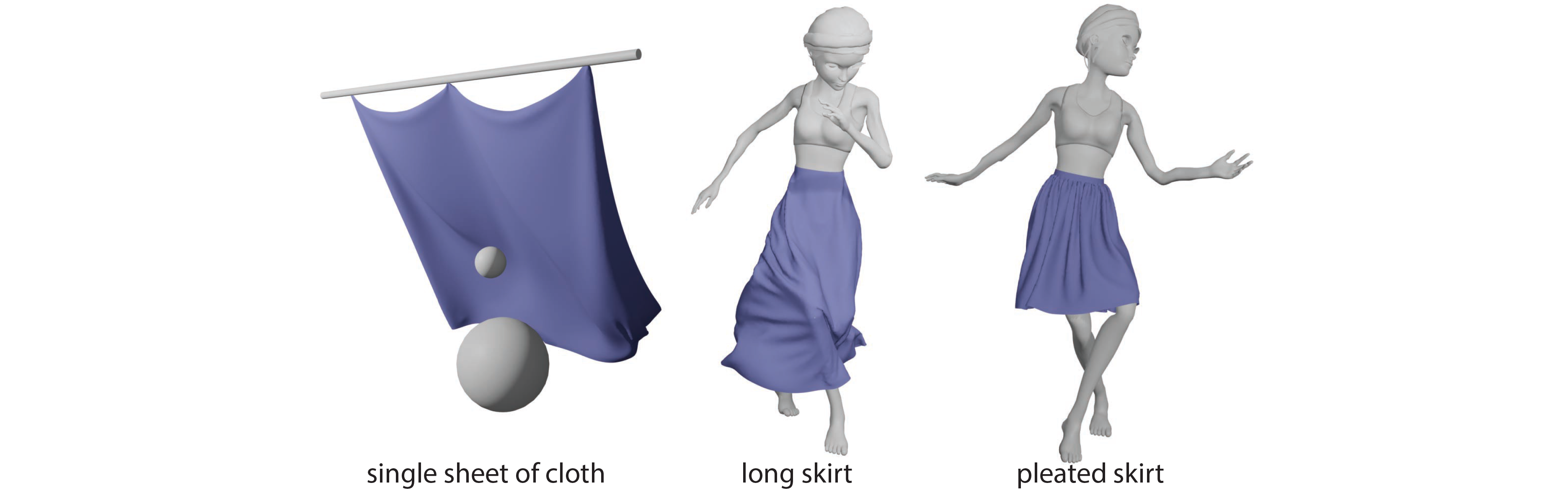
Our base training data is simulated from a single sheet of cloth interacting with two balls and blowing wind. We also simulate a long skirt and a pleated skirt under different body motions. For each training example, we simulate both a coarse and a fine resolution mesh and obtain the corresponding normal map pairs.
Results Gallery

We evaluate our method both on coarse garment simulations and garments deformed by linear blend skinning. Our method can generalize across different garment types and motions. The materials for the simulated garments are as follows: t-shirt (knit terry), pants (wool melton), skirt (silk chamuse), skirt laces (silk chiffon), hanfu (silk chamuse), hood (denim lightweight), pants (knit terry), vest (wool melton). For the two skinning based examples, our material classification network predicts them respectively as silk chiffon (the red) and knit terry (the yellow). Please see supplementary video.
Bibtex
@inproceedings{zhang2021deep, title={Deep detail enhancement for any garment}, author={Zhang, Meng and Wang, Tuanfeng and Ceylan, Duygu and Mitra, Niloy J}, booktitle={Computer Graphics Forum}, volume={40}, number={2}, pages={399--411}, year={2021}, organization={Wiley Online Library} }
Acknowledgements
The authors would like to thank the anonymous reviewers for their constructive comments; Marvelous Designer for their generous support; Adobe Fuse for the virtual bodies and Mixamo for the motion sequences; and the various artists for sharing creative garment models on Mixamo, Microsoft rocket box, and Marvelous Designer store. This work was partially supported by the ERC SmartGeometry grant and gifts from Adobe Research.